Can Activity Trackers Create Better Clinical Trials?

By Ed Miseta, Chief Editor, Clinical Leader
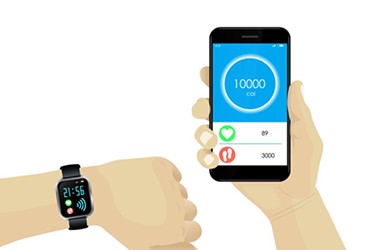
I recently reported on data collected by Fitbit activity trackers. The company has compiled 150 billion hours of data on the resting heart rate (RHR) of tens of millions of people around the world who used a Fitbit. Some of the results were what you might expect, but there were some surprises in the data as well. What I wondered as I reviewed that data was how the information could help researchers, especially cardiologists, conduct better clinical trials on heart patients in the future.
To gain some insights, I reached out to Dr. Roger Mills, who has spent his entire career in medicine working as a physician, cardiologist, professor, and drug developer. I asked Mills if having access to this information would allow the industry to produce better trials and possibly even predict cardiac events such as stroke or heart attack. While the questions seem simple, the answers are much more complicated.
First off, billons of hours of data collected on tens of millions of patients, along with their sex, age, height, weight, and location, is what researchers would refer to as big data. “It’s an unbelievable number of data points and possible associations,” says Mills. “Clearly this is fodder for very bright people with very BIG computers.”
Valid And Interpretable?
To answer my questions, Mills opted to first look at two other important questions. The first thing he feels we need to consider is whether or not the data are valid.
“We have a fair amount of information about Fitbit technology,” states Mills. “If we look at activity level, the technology seems quite reliable when recording the number of steps of users who do not have mobility problems. The validity is lower for the calculation of energy expenditure.”
For the data relating to heart rate, Mills points to data from four studies published this year. One of those studies indicated commercial wearable devices “may be useful in obtaining an estimate of heart rate for everyday activities and general exercise.” Another study noted that, of the brands currently available, the five most often used in research projects are Fitbit, Garmin, Misfit, Apple, and Polar. Fitbit is used in twice as many validation studies as any other brands and is registered in ClinicalTrials.gov studies 10 times as often as other brands. That makes Fitbit the most widely studied and used technology. The study also concluded that advances in device quality will continue to offer new opportunities for research.
However, there were issues related to the data. Mills notes technical problems include difficulty with sensing atrial tachycardias and the fact that data for some individuals may be far from accurate. A published paper by Benedetto, Caldato, and Bazzan titled, “Assessment of the Fitbit Charge 2 for Monitoring Heart Rate” found the Fitbit underestimates the heart rate. The mean bias in measuring heart rate was a modest -5.9 bpm. Even so, the precision of individual measurements between the Fitbit Charge 2 and an electrocardiograph (measured by having an individual ride a stationary bike for 10 minutes) were wide. The limits of agreement, which indicate the precision of individual measurements, were wide (+16.8 to -28.5 bpm). This indicates that an individual heart rate measure could plausibly be underestimated by almost 30 bpm. In terms of validity of data, it seems Fitbit devices could do better.
Next, Mills asked if the data collected was interpretable. His answer to that question is a firm “Maybe.”
“We are certainly beginning to see application of advanced computer technology to medical problems, and I anticipate that we will see some interesting associations and patterns as the Fitbit data are parsed,” he says. “Much of this may be confirmatory, but some will be novel. Remember, these are observational data. We can’t, for instance, say that a statistically significant association in such a huge dataset implies something about causality. Furthermore, we must also remember that whatever we learn is limited to people who, for one reason or another, decided to purchase a Fitbit and also decided to wear it for a period of time. What was behind those decisions? There are confounders that are simply too numerous to count.”
Can We Impact Trials?
Knowing what we do about the Fitbit data, can we use it, or these mobile devices, to improve trials for cardiac patients? Mills believes we need to make sure our clinical trials in heart disease are testing the correct question, with reasonable methods and statistical power, in the appropriate population. The last point is where he sees the possibility of some real utility from these large datasets.
“Predicting events for a given individual is unlikely,” he says. “However, defining a population where endpoint events occur often enough to have statistical power with a reasonably sized trial, but not so often that the events are essentially inevitable (so that it’s impossible to show a treatment effect) is one of the critical elements in designing a successful trial. If big data have real promise for trial design, it’s likely to be in helping us improve inclusion and exclusion criteria so that we can demonstrate efficacy and safety more efficiently.”
First, researchers have to be able to gather the data they need. Devices such as a Fitbit can measure both duration and intensity of activity. At the same time, these noninvasive and objective measurement devices do not require participants to undergo training and can be worn without interfering with an individual’s daily activities. Fitness trackers can also precisely measure low levels of activity. Therefore, they can do a good job of presenting researchers with insights into the daily activity levels of wearers.
Once we have that data, researchers need to figure out how to analyze it. They also need to figure out if there is an appropriate endpoint. Mills believes there is. He points to one published study from January 2015 in the Journal of the American Heart Association (Khan, Kunutsor, and Kalogeropoulos et al.) titled “Resting Heart Rate and Risk of Incident Heart Failure: Three Prospective Cohort Studies and a Systematic Meta-Analysis.”
The study found in a pooled random effects meta-analysis of seven population-based studies (43,051 participants and 3,476 heart failure events), the overall hazard ratio comparing top versus bottom fourth of RHR was 1.40, meaning there is a non-linear association between RHR and incident heart failure. This could help define a population for clinical researchers to study. Mills does note that further research is needed to fully understand the physiologic foundations of this association.
More work still needs to be done, and trials may require better devices than a Fitbit activity tracker. But the data seems to point to a future for activity tracking devices in clinical trials.